6th April 2020
I recently “visited some” museum websites to determinate which content management system or backend that powers them. Now I have published my dataset with over 370 Swedish museum websites (excluding those museums who uses central CMS solutions (such as hembygd.se or municipality websites)).

Almost two thirds are powered by WordPress (general CMS marketshare estimated between 35-50%). Drupal, SiteVision, and Joomla! comes there after all powering less than a dozen sites.
This homogeneous situation could have interesting applications but I will save those thoughts for a future post. For now you can get the dataset and read more about it over at Figshare. It's licensed under CC0.
18th February 2020
Nationalmuseum, the national gallery of Sweden has had an API for about two and a half years (I think),but it has yet to have any public documentation. So I decided to write down its features and some random tips.
Capabilities
The API is able to return specific objects as well as returning all objects through pagination. Many objects also link to their external IIIF manifest.
Retrieving an object
https://api.nationalmuseum.se/api/objects/4000
Check.
The API has two parameters for pagination page
and limit
. limit
sets the number of objects you get per page, the default is 50 and allowed values range from 1-100.
Get the 100-200 objects:
https://api.nationalmuseum.se/api/objects?page=2&limit=100
The response also returns you the URLs for the previous and next pages taking the limit parameter into account.
Random notes
The URLs to items can seem daunting when you browse Nationalmuseums online collection but there is an alternative that’s easy to link to with the identifier you get from the API:
http://collection.nationalmuseum.se/eMP/eMuseumPlus?service=ExternalInterface&module=collection&viewType=detailView&objectId=24342
A great way to find interesting objects in the collection and connect it to other sources is to ask Wikidata:
Objects in Wikidata with Nationalmuseum IDs
Finally, for some inspiration, you could check out the Nationalmuseum VIKUS Viewer instance.
7th February 2020
I haven’t done a weekly reading list on this blog since high school; this week I did for a mysterious unknown reason. Alternative title: Links with random mumblings.
GLAM and Museum Tech
Accessibility
- Why You Should Choose HTML5 <article> Over <section> – Even if the title doesn’t teach you something new, the article will still show an actual useful use of the <section> element.
- HTML attributes to improve your users’ two factor authentication experience https://www.twilio.com/blog/html-attributes-two-factor-authentication-autocomplete – As often with web accessibility/usability things, what’s details to developers aren’t necessarily details for your users.
- Making Memes Accessible – Intresting problem; I’m however sligthly sceptical against the implementation. Partial image hashing could be a better solution to matching images maybe?
Other
19th January 2020
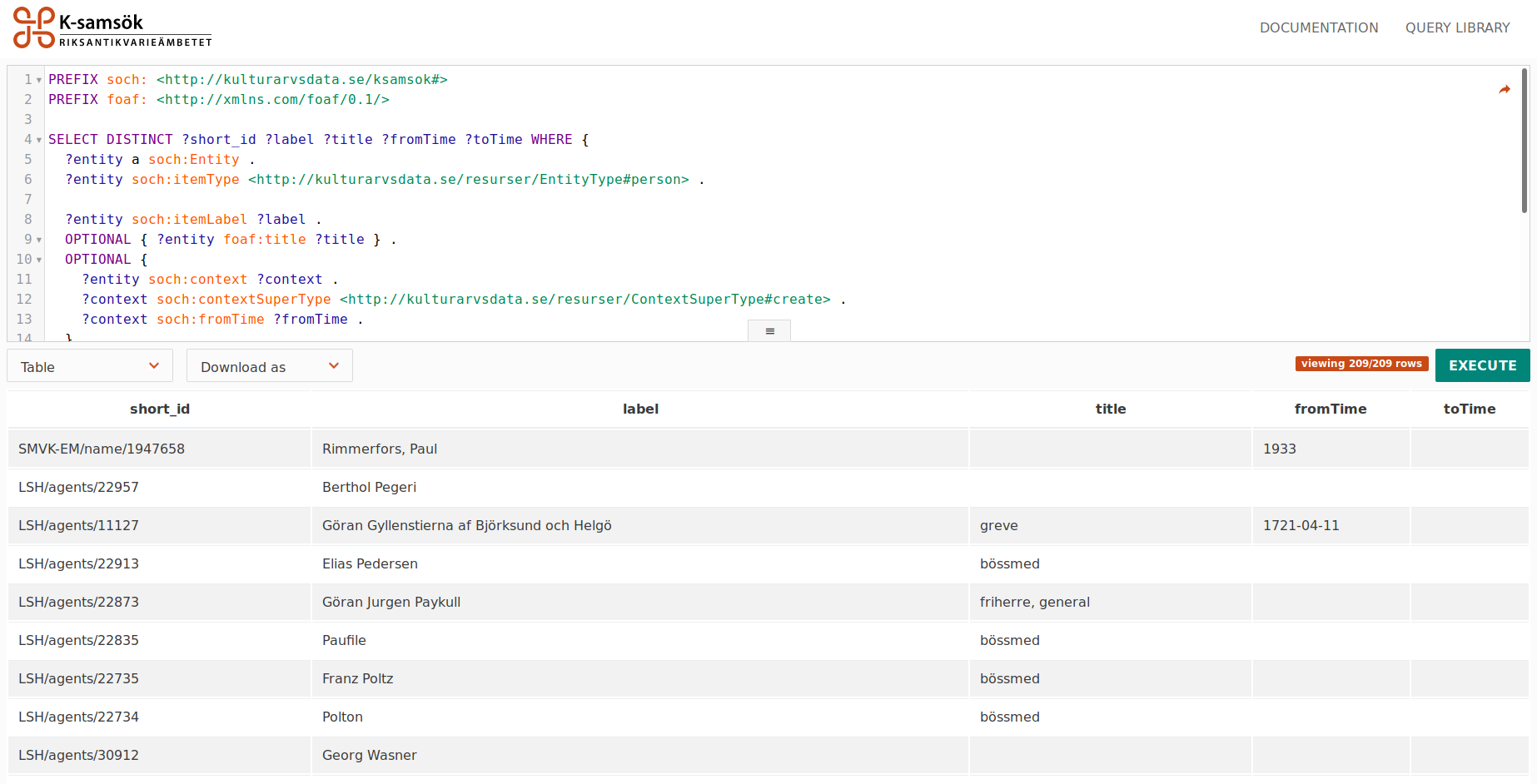
About a year ago I made a custom SPARQL editor for the Swedish Open Cultural Heritage (SOCH/K-samsök) LOD platform since then it has served me, both at home and at the office. Now I’m hosting an instance for anyone to use. It was built both to aid me (and others) working with the over 8 million RDF records in SOCH as well as to be a prof of concept and reference for future work.
The editor comes with a ton of features including:
- Autocompletion of the entire SOCH ontology
- Autocompletion of some external endpoints, including Wikidata and Europeana
- Result visualizations, including a table, an image grid and a pie chart
- Integration with a query library
- Shareable queries
- A resizable code editor
- An interactive tour of its GUI
There is no official SPARQL endpoint for SOCH so the first time you uses the editor you will be asked to enter your own endpoint or a third party one.
You can find the editor here and yes it’s inspired by the WDQS GUI.
How to setup a SPARQL endpoint with SOCH data?
First I usually bulk download RDF records with SOCH download CLI. Secondly I load these into Fuseki (super easy to setup, but somewhat low performance if you load all of SOCH into it) or Blazegraph (trickier to setup but great performance).